In what ways can AI be used to improve longevity research and/ or medicine?
Image credit: Photos by Markus Spiske and Pixabay from Pexels
Please leave the feedback on this challenge
Necessity
Is the problem still unsolved?
Conciseness
Is it concisely described?
Bounty for the best solution
Provide a bounty for the best solution
Bounties attract serious brainpower to the challenge.
Creative contributions
Deep Aging Clocks that estimate an individual's biological age
[1]Zhavoronkov, A., Bischof, E. & Lee, KF. Artificial intelligence in longevity medicine. Nat Aging 1, 5–7 (2021). https://doi.org/10.1038/s43587-020-00020-4
[2]Zhavoronkov A, Mamoshina P. Deep Aging Clocks: The Emergence of AI-Based Biomarkers of Aging and Longevity. Trends Pharmacol Sci. 2019 Aug;40(8):546-549. doi: 10.1016/j.tips.2019.05.004. Epub 2019 Jul 3. PMID: 31279569.
Please leave the feedback on this idea
Deep algorithms for early detection of diseases
[1]Ying-Chen Claire Hou, Hung-Chun Yu, Rick Martin, Elizabeth T. Cirulli, Natalie M. Schenker-Ahmed, Michael Hicks, Isaac V. Cohen, Thomas J. Jönsson, Robyn Heister, Lori Napier, Christine Leon Swisher, Saints Dominguez, Haibao Tang, Weizhong Li, Bradley A. Perkins, Jaime Barea, Christina Rybak, Emily Smith, Keegan Duchicela, Michael Doney, Pamila Brar, Nathaniel Hernandez, Ewen F. Kirkness, Andrew M. Kahn, J. Craig Venter, David S. Karow, C. Thomas Caskey. Precision medicine integrating whole-genome sequencing, comprehensive metabolomics, and advanced imaging. Proceedings of the National Academy of Sciences Feb 2020, 117 (6) 3053-3062; DOI: 10.1073/pnas.1909378117
Please leave the feedback on this idea
Please leave the feedback on this idea
Please leave the feedback on this idea
Machine learning-powered molecular docking tools to predict the side-effects of novel anti-aging therapies
[1]Sukyung Seo, Taekeon Lee, Mi-hyun Kim, Youngmi Yoon, "Prediction of Side Effects Using Comprehensive Similarity Measures", BioMed Research International, vol. 2020, Article ID 1357630, 10 pages, 2020. https://doi.org/10.1155/2020/1357630
[2]Xiaoyi Guo, Wei Zhou, Yan Yu, Yijie Ding, Jijun Tang, Fei Guo, "A Novel Triple Matrix Factorization Method for Detecting Drug-Side Effect Association Based on Kernel Target Alignment", BioMed Research International, vol. 2020, Article ID 4675395, 11 pages, 2020. https://doi.org/10.1155/2020/4675395
[3]Haiyan Liang, Lei Chen, Xian Zhao, Xiaolin Zhang, "Prediction of Drug Side Effects with a Refined Negative Sample Selection Strategy", Computational and Mathematical Methods in Medicine, vol. 2020, Article ID 1573543, 16 pages, 2020. https://doi.org/10.1155/2020/1573543
Please leave the feedback on this idea
An integrative multifactorial AI-powered aging metric
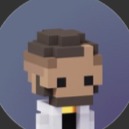
[1]Mamoshina, P., Kochetov, K., Putin, E., Cortese, F., Aliper, A., Lee, W.-S., Ahn, S.-M., Uhn, L., Skjodt, N., Kovalchuk, O., et al. (2018). Population Specific Biomarkers of Human Aging: A Big Data Study Using South Korean, Canadian, and Eastern European Patient Populations. Journals Gerontol. Ser. A 73, 1482–1490.